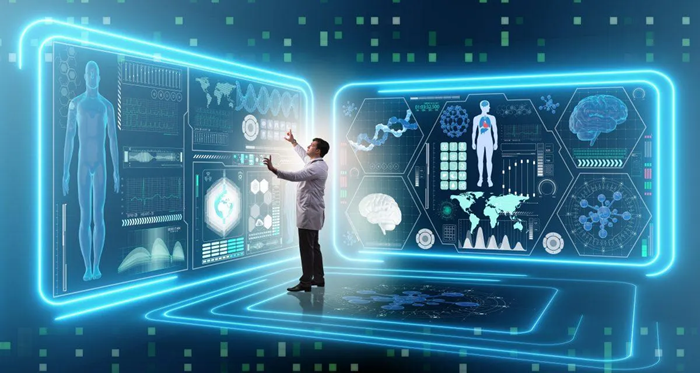
Audio data collection is a pivotal step in the development of robust and accurate machine learning models for diverse applications ranging from speech recognition to sentiment analysis. This in-depth guide delves into the multifaceted world of audio data collection, encompassing methodologies, best practices, challenges, and future trends, an indispensable phase in the realm of AI development, that serves as the lifeblood for training cutting-edge machine learning models. This comprehensive guide delves into the multifaceted landscape of audio data collection, exploring methodologies, ethical nuances, technical challenges, best practices, and futuristic trends shaping the domain.
Significance of Audio Data Collection
In an increasingly voice-centric technological landscape, the importance of high-quality audio datasets cannot be overstated. From powering virtual assistants to facilitating voice-driven interactions, audio data collection serves as the bedrock for training AI models. Robust datasets that encompass a broad spectrum of languages, accents, and environmental conditions are essential for fostering inclusive and adaptable AI systems. The proliferation of voice-powered devices, automated speech recognition systems, and conversational AI underscores the paramount significance of high-quality audio datasets. These datasets underpin the accuracy, adaptability, and inclusivity of machine learning models, enabling seamless interactions and intuitive understanding of human language nuances.
Methodologies for Audio Data Collection
- Recording Devices and Environments: Selecting appropriate recording devices, whether microphones, smartphones, or specialized equipment, and identifying diverse environments for capturing audio data, such as quiet rooms, outdoor settings, or noisy environments, are critical initial considerations.
- Sampling Rates, Formats, and Bit Depths: Understanding the intricacies of audio formats (e.g., WAV, MP3, FLAC), sampling rates, and bit depths to ensure optimal quality preservation and compatibility across platforms.
- Acquisition of Diverse Audio Samples: Explore strategies to collect diverse audio samples encompassing various languages, dialects, accents, and environmental conditions. This includes capturing data in controlled settings, real-world scenarios, and under different acoustic conditions to ensure robustness in model training.
- Annotation Guidelines and Metadata: Crafting comprehensive annotation guidelines that encompass essential metadata (speaker information, emotional cues, background noises) to enrich the dataset for precise model training.
- Data Diversity and Volume: Ensuring diversity in collected data by capturing variations in accents, languages, speech patterns, and scale, while also managing the volume of data efficiently for storage, processing, and scalability.
- Metadata Enrichment and Annotation: Elaborate on the significance of metadata enrichment, including speaker identification, emotional cues, environmental context, and linguistic markers. Discuss annotation methodologies like phonetic transcription, semantic tagging, and sentiment analysis to enhance dataset granularity.
Best Practices for Audio Data Collection
- Ethical Considerations and Informed Consent: Prioritizing ethical practices by obtaining informed consent from participants and ensuring compliance with data privacy regulations.
- Quality Assurance and Preprocessing: Implementing rigorous quality checks, noise reduction, normalization techniques, and preprocessing methodologies to maintain high-quality audio datasets.
- Data Security and Storage: Adhering to robust data security measures for storage, transmission, and access control to safeguard sensitive audio information.
- Metadata Enrichment and Labeling: Gathering comprehensive metadata alongside audio recordings and employing efficient labeling strategies to facilitate supervised learning tasks while maintaining efficiency.
Challenges and Considerations
- Bias Mitigation and Representation: Addressing inherent biases in collected data to achieve fair representation across demographics and avoid perpetuating biases in machine learning models. Examine strategies to address biases in collected data, ensuring representation across diverse demographics and cultures to prevent algorithmic bias.
- Annotator Subjectivity and Consistency: Mitigating annotator subjectivity by establishing standardized annotation protocols to ensure consistency and accuracy in labeling. Elaborate on measures to mitigate annotator subjectivity through inter-rater reliability tests, training, and guidelines, ensuring consistent and accurate labeling.
- Scalability and Management: Overcoming challenges associated with managing large volumes of audio data by employing scalable storage solutions and efficient data management practices.Explore scalable storage solutions, cloud-based architectures, and data management frameworks to handle large volumes of audio data efficiently.
Future Trends and Innovations
- Active Learning and Sampling Strategies: Incorporating active learning paradigms and advanced sampling strategies to optimize the data collection process by iteratively selecting samples that maximize model learning. Discuss active learning paradigms and adaptive sampling strategies leveraging uncertainty estimation and reinforcement learning to optimize the data collection process.
- Synthetic Data Generation and Augmentation: Exploring synthetic data generation methods to augment real-world data, enhancing dataset diversity and scalability for improved model performance. Analyze the role of generative models such as GANs (Generative Adversarial Networks) for synthesizing realistic audio data, augmenting existing datasets to enhance diversity and volume.
- Privacy-Preserving Techniques and Federated Learning: Embracing privacy-preserving techniques like federated learning to train models without centralized data storage, ensuring data privacy and security. Delve into advanced privacy-preserving techniques like differential privacy, homomorphic encryption, and federated learning to train models while respecting user privacy.
Challenges and Mitigation Strategies
- Annotator Reliability and Consistency: Address challenges related to annotator variability and the potential for errors in crowdsourced data. Propose strategies like redundancy in annotations and consensus-based approaches to ensure accuracy.
- Ethical Considerations: Discuss the importance of maintaining ethical standards, such as ensuring participant consent, data privacy, and fair compensation for contributors, while adhering to regulatory guidelines in crowdsourced data collection.
Integration with Existing Data Collection Practices
- Hybrid Approaches: Explore the integration of crowdsourced data with in-house or expert-annotated datasets to create comprehensive. And diverse audio datasets with improved accuracy and coverage.
- Continuous Feedback Loop: Highlight the iterative nature of crowdsourced data collection. Allowing for continuous improvements based on feedback and ongoing quality assessment mechanisms.
Benefits and Considerations
- Scalability and Speed: Highlight how crowdsourcing enables scalability by harnessing the collective efforts of a large number of contributors. Allowing for the rapid accumulation of vast audio datasets.
- Quality Control Mechanisms: Discuss quality control measures such as consensus-based annotation, and worker qualifications. And periodic checks to maintain data quality and reliability in crowdsourced tasks.
- Diverse Contributors and Global Reach: Emphasize the advantage of accessing a diverse pool of contributors worldwide. Enabling the collection of audio data that reflects varied linguistic nuances, accents, and cultural contexts.
- Future Potential and Innovations
- AI-Driven Quality Assurance: Discuss the potential for integrating AI algorithms to assist in quality control processes. Automating annotation verification, and ensuring higher accuracy and consistency.
- Community Engagement and Collaboration: Explore avenues for engaging contributors as a community, fostering collaboration. And incentivizing ongoing participation in audio data collection tasks.
Conclusion
Audio data collection stands as a cornerstone in the development of AI applications that rely on voice and audio interactions. By following meticulous methodologies, adhering to ethical principles, addressing challenges, and embracing emerging trends. Practitioners can curate high-quality, diverse audio datasets that serve as the foundation for innovative and ethically responsible AI solutions.